Digital transcriptome subtraction
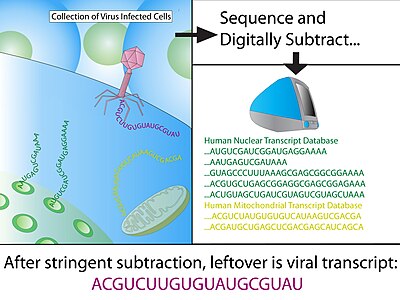
Digital transcriptome subtraction (DTS) is a
History
Using computational subtraction to discover novel pathogens was first proposed in 2002 by Meyerson et al.
In 2007, the term "Digital Transcriptome Subtraction" was coined by the Chang-Moore group,[4] and was used to discover Merkel cell polymavirus in Merkel-cell carcinoma.[1]
Simultaneously to the MCV discovery, this approach was used to implicate a novel arenavirus as cause of fatality in a case where three patients died of similar illnesses shortly following organ transplantations from a single donor.[5]
Method

Construction of cDNA library
After treatment with
Sequencing and quality control
The cDNA library must be sequenced to great depth (i.e. number of clones sequenced) in order to detect a theoretical rare pathogen sequence (Table 1), especially if the foreign sequence is novel. Chang-Moore recommend a sequencing depth of 200,000 transcripts or greater using multiple sequencing platforms.[1]
% Viral | 5,000 clones | 10,000 clones | 20,000 clones | 50,000 clones |
---|---|---|---|---|
0.001% | 4.9% | 9.5% | 18.1% | 39.3% |
0.01% | 39.3% | 32.2% | 86.5% | 99.3% |
0.02% | 63.2% | 86.5% | 98.2% | >99.995% |
0.03% | 77.7% | 95.5% | 99.8% | >99.995% |
0.04% | 86.5% | 98.2% | >99.995% | >99.995% |
0.1% | 99.3% | >99.995% | >99.995% | >99.995% |
Stringent quality control are then applied to the raw sequences to minimize false-positive results. The initial quality screen uses several general parameters to exclude ambiguous sequences, leaving behind a dataset of high-fidelity (Hi-Fi) reads.
- Low Phred scorecutoff is used to remove low-quality end sequences. Typically, a Phred score cutoff of 20 or 30 is used to ensure 99%-99.9% accuracy in each base-calling.
- Vector and adaptor removal.
- Low complexity - complexity score of a sequence reflects number of identical bases in a series (homo-polymers) such as poly-dT or poly-dA.
- Human repetitive DNA.
- Length - parameter is dependent on the optimized read length specific to the sequencing technologythat was used.
- E. coligenome sequences.
BLAST to host genome
Using MEGABLAST, Hi-Fi reads are then matched to sequences in annotated databases and any positive matches are then subtracted from the dataset. Minimum hit length for a positive match of human sequence is typically 30 consecutive identical bases, which equates to a BLAST score of 60; generally, the remaining sequence is BLAST again with less stringent parameters to allow for slight mismatches (1 in 20 nucleotide). The vast majority of sequences (>99%) should be removed from the dataset at this stage.
Subtracted sequences typically include:
- Reference human transcriptome - eliminates any known human transcripts from expression library sets.
- Reference human genome - eliminates genes that have been missed by the annotation process and any contaminating genomic sequences during cDNA library construction.
- Mitochondrial DNA - mitochondrial DNA are highly abundant and polymorphic due to rapid mutation rate.
- Immunoglobulin region- The immunoglobulin loci is highly polymorphic and would otherwise yield false-positive due to poor alignment to the reference genome.
- Other vertebrate sequences
- Unannotated sequences
Analysis of "non-host" candidates
Alignment to pathogen databases
After stringent rounds of subtraction, the remaining sequences are clustered into non-redundant contigs and aligned to known pathogen sequences using low-stringency parameters. As pathogen genomes mutates quickly, nucleotide-nucleotide alignments, or
De novo assembly
In cases where alignment to known pathogens is uninformative or ambiguous, contigs of candidate sequence can be used as templates for
Validation of pathogen
Once a putative pathogen has been identified in the high-throughput sequencing data, it is imperative to validate the presence of pathogen in infected patients using more sensitive techniques, such as:
- RT-PCR and derivative methods, including 3'- and 5'-RACEto confirm the existence of pathogen mRNA.
- Immunohistochemistry using antibodies to related pathogen to determine existence the pathogen in tissues.
- Serological tests to measure pathogen-specific antibody titer.
- Bacterial culture/viral culture, which is considered as the gold standardin laboratory diagnosis.
Applications
The primary application for DTS lies in identification of pathogenic viruses in cancer.[1][4] It can also be used to identify viral pathogens in non-cancer related disease.[5] Future clinical applications could include the use of DTS on a routine basis in individuals. DTS could also apply to
Advantages
- Requires no prior knowledge about pathogen sequence.[8]
- Can identify previously unassociated, potentially treatable pathogens.
- Uses already available molecular methods and resources.
Disadvantages
- Identifies the presence of pathogen but does not establish causal link to disease.Koch's postulate and Bradford Hill criteria.
- Must have a highly reliable, complete reference transcriptome for the organism being studied.[8]
- Lack of foreign sequence identification cannot entirely exclude a pathogenic foreign body.[8]
References
- ^ PMID 18202256.
- ^ S2CID 21842679.
- ^ PMID 12659816.
- ^ PMID 17686852.
- ^ PMID 18256387.
- ^ Chang Y, Moore PS. "New Pathogen Discovery: Digital Transcriptome Subtraction". Archived from the original on 25 January 2010. Retrieved 1 March 2012.
- S2CID 14013425.
- ^ PMID 18368124.